Chapter 10: NumPy - Our data container
The evaluation of scientific data, i.e. measurement data, is almost always about the evaluation of numbers. This applies, e.g., to LFP time series, patch-clamp recordings or multi-fluorescence images.
The mathematical functions of pure Python are limited. Anything beyond basic operations (+, -, /, *) has to be programmed by ourselves. We can of course do that – or: We use one of the many packages where others have already done the work for us. Such a useful package is NumPy (numpy.org/doc/stable/).
The NumPy logo. Image source: commons.wikimedia.org, license: CC BY-SA 4.0
IntroductionPermalink
Let’s have a first look:
import numpy as np
# let's create our first NumPy-array:
array_1 = np.arange(10)
array_2 = np.array([1, 2, 3])
print(f"array_1: {array_1}")
print(f"array_2: {array_2}")
array_1: [0 1 2 3 4 5 6 7 8 9]
array_2: [1 2 3]
The import
command makes all functions within the NumPy package available for our own program. These functions can be called by np.FUNCTION(...)
, e.g. np.mean(array_1)
:
print(f"np.mean(array_1): {np.mean(array_1)}")
np.mean(array_1): 4.5
The np.arange(start,stop,step)
command creates a so-called NumPy-array similar to the range
command, but with the advantage that we can directly access the array (to achieve the same with the range
command: list(range(10))
). And we now can create arrays with floating point numbers:
array_3 = np.arange(0, 5, 0.5)
print(f"array_1: {array_3}")
array_1: [0. 0.5 1. 1.5 2. 2.5 3. 3.5 4. 4.5]
The np.array([1, 2, 3])
command converts any given list (or other array-like object) into a NumPy array.
One main advantage of a NumPy array is, that one can directly call basic NumPy functions via array.mean()
instead of np.mean(array)
(while both is valid):
print(f"array_1.mean(): {array_1.mean()}")
array_1.mean(): 4.5
Some further examples:
print(f"array_1.std(): {array_1.std()}")
print(f"array_1.max(): {array_1.max()}")
print(f"array_1.min(): {array_1.min()}")
print(f"array_1.sum(): {array_1.sum()}")
array_1.std(): 2.8722813232690143
array_1.max(): 9
array_1.min(): 0
array_1.sum(): 45
Not all NumPy functions can be accessed this way:
print(f"array_1.median(): {array_1.median()}")
AttributeError...
print(f"np.median(array_1): {np.median(array_1)}")
np.median(array_1): 4.5
Useful NumPy functionsPermalink
Command | Description |
---|---|
Creating and manipulating arrays: | |
np.arange(number) |
creates an array of evenly spaced values, e.g. np.arange(3) creates an array of the numbers 1, 2, 3 |
np.array([1,2,3]) |
creates an array, e.g., of the numbers 1, 2, 3 |
np.shape(array) |
get the shape (dimensions and lengths) of array |
array.dtype |
get the type of array (integer?, float?, …) |
array.reshape |
reshapes array, e.g. from shape (6) to (2,3) |
np.transpose or array.T |
transpose array |
np.append(array1, array2) |
append array1 to array2 |
np.concatenate((array1, array2)) |
concatenate array1 and array2 |
np.insert(array, index, value) |
insert value at index-position of array |
np.delete(array, index) |
delete entry at index-position of array |
Useful statistical functions: | |
np.mean(array) |
calculate the average of array |
np.median(array) |
calculate the median of array |
np.std(array) |
calculate the standard deviation of array |
np.sum(array) |
calculate the sum of array |
np.cumsum(array) |
calculate the cumulative sum of array |
np.max(array) |
calculate the maximum of array |
np.min(array) |
calculate the minimum of array |
Useful mathematical functions: | |
np.sqrt(array) |
calculate the square root of array |
np.square(array) |
calculate the square of array |
np.abs(array) |
calculate the element-wise absolute values of array |
np.exp(array) |
calculate the exponentiation of array |
np.log(array) |
calculate the natural logarithm of array |
np.sin(array) |
calculate the sine of array |
np.cos(array) |
calculate the cosine of array |
np.round(array) |
round array |
np.floor(array) |
floor of the array |
np.ceil(array) |
ceiling of the array |
np.sort(array) |
sort array |
np.nan_to_num(array) |
replace NaN (Not a Number) with zero and infinity with large finite numberization |
You can find a broader overview of NumPy functions, e.g., on the Datacamp ꜛ NumPy cheat sheet ꜛ.
Of course, you can also construct your own functions using existing NumPy functions, e.g.:
""" Let's construct a simple function, that searches the closest
value within an NumPy for a given search value:
"""
import numpy as np
# define the function:
def find_nearest(input_array, search_number):
"""
Simple function to search for the closest
value within a given NumPy array.
input_array: input array within we search
search_number: integer or floating point
number to search for in
input_array
Caution: This function is not bullet-proof: It does
not return multiple occurrences of the found
closest(s) value(s).
"""
array = np.asarray(input_array)
idx = (np.abs(array - search_number)).argmin()
return array[idx], idx
# apply the function to a test array:
array_test = np.array([0, 5, 5, 6, 6, 3, 9])
print(array_test)
print(find_nearest(array_test, 5.6))
[0, 5, 5, 6, 6, 3, 9]
6, 3
On array dimensionalityPermalink
One very useful NumPy command is np.shape(array)
or array.shape
, which returns the size and the shape (dimensions) of a given array:
print(f"array_1.shape(): {array_1.shape}")
array_1.shape(): (10,)
The output (10,)
indicated, that array_1
has only one axis, i.e. it is one-dimensional, with 10 entries on that axis. We can of course create NumPy arrays with higher dimensions:
# 2D array (e.g. an image):
array_4 = np.array([(1, 2, 3),
(4, 5, 6),
(7, 8, 9),
(7, 8, 9)])
print(f"array_4:")
print(array_4)
print(f"array_4.shape(): {array_4.shape}")
array_4:
[[1 2 3]
[4 5 6]
[7 8 9]
[7 8 9]]
array_4.shape(): (4, 3)
# 3D array (e.g. an image stack):
array_5 = np.array([[(1,2,3), (4,5,6)],
[(3,2,1), (4,5,6)]])
print(f"array_5:")
print(array_5)
print(f"array_5.shape(): {array_5.shape}")
array_5:
[[[1 2 3]
[4 5 6]]
[[3 2 1]
[4 5 6]]]
array_5.shape(): (2, 2, 3)
Visual overview of NumPy array dimensionalities:
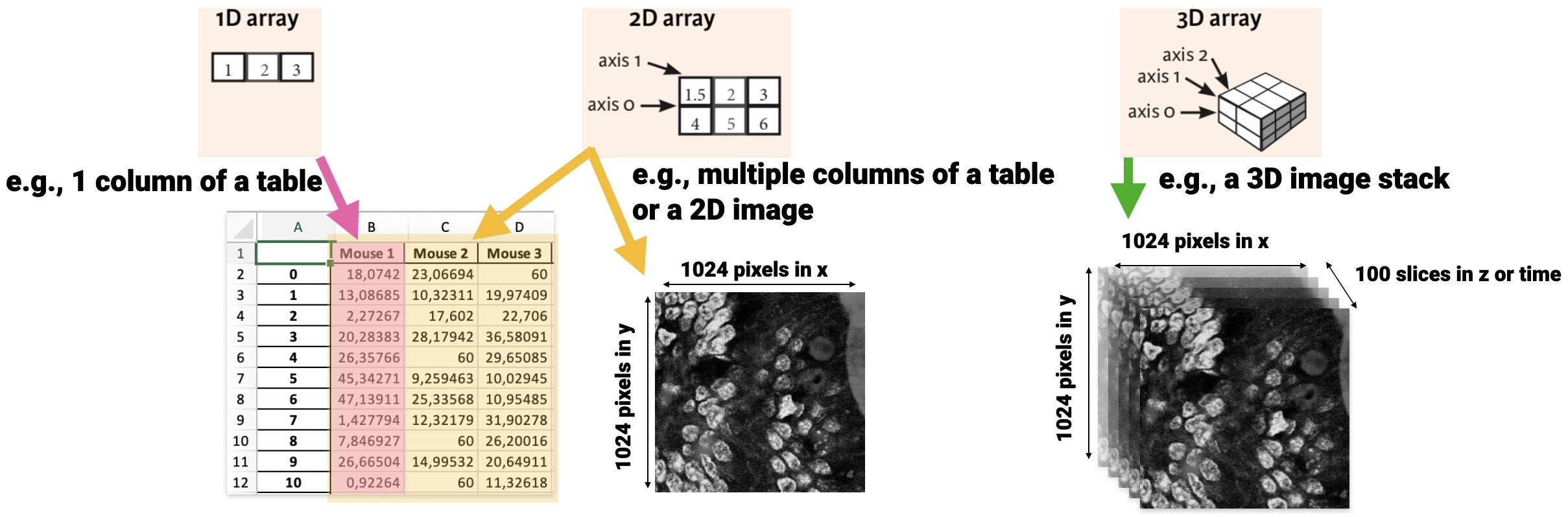
Useful helper functions for array generationPermalink
NumPy brings some little helper functions to create n-dimensional arrays (sometimes helpful in prototyping and testing your programs or to understand a program):
# let NumPy create some arrays:
Z = np.zeros((3,4))
print(f"Z:")
print(Z)
Z:
[[0. 0. 0. 0.]
[0. 0. 0. 0.]
[0. 0. 0. 0.]]
O = np.ones((3,4))
print(f"O:")
print(O)
O:
[[1. 1. 1. 1.]
[1. 1. 1. 1.]
[1. 1. 1. 1.]]
L = np.linspace((1,21),(5,25),5)
""" creates a 2D array; 1st column ranges from 1 (of (1,11))
to 5 (of (5,15)), automatically spaced, so that we get
5 (last number in the command) equally spaced increasing
numbers. """
print(f"L:")
print(L)
L:
[[ 1. 21.]
[ 2. 22.]
[ 3. 23.]
[ 4. 24.]
[ 5. 25.]]
np.random.seed(1)
R = np.random.random((4,3))
# Creates some random numbers. The command np.random.seed(1)
# fixes the machine internal random state for reproducibility.
print(f"R:")
print(R)
R:
[[4.17022005e-01 7.20324493e-01 1.14374817e-04]
[3.02332573e-01 1.46755891e-01 9.23385948e-02]
[1.86260211e-01 3.45560727e-01 3.96767474e-01]
[5.38816734e-01 4.19194514e-01 6.85219500e-01]]
Indexing and slicingPermalink
Indexing and slicing of NumPy arrays works similar as for lists:
print(f"shape of R: {R.shape} (4 rows, 3 columns)")
shape of R: (4, 3) (4 rows, 3 columns)
print(f"R[0,0]:")
print(R[0,0]) # Note, R is 2D and needs therefore two
# index-values:
# first: row-index, second: column-index
R[0,0]:
0.417022004702574
print(f"R[:,0]:")
print(R[:,0])
R[:,0]:
[0.417022 0.30233257 0.18626021 0.53881673]
print(f"R[1:4,0]:")
print(R[1:4,0])
R[1:4,0]:
[0.30233257 0.18626021 0.53881673]
Also the output of the shape
command is indexable:
print(f"R.shape[0]: {R.shape[0]} (4 rows)")
print(f"R.shape[1]: {R.shape[1]} (3 columns)")
R.shape[0]: 4 (4 rows)
R.shape[1]: 3 (3 columns)
Exercise 1Permalink
Write a script, that defines the 2D NumPy array L
as defined above/as follows:
L = np.linspace((1,11),
(5,15),
5)
Iterate L
- over each row. Calculate and print the average of each row.
- over each column. Calculate and print the average of each column.
# Your solution here:
L:
[[ 1. 21.]
[ 2. 22.]
[ 3. 23.]
[ 4. 24.]
[ 5. 25.]]
averages over rows:
11.0
12.0
13.0
14.0
15.0
averages over columns:
3.0
23.0
Toggle solution
# Solution 1:
print("L:")
print(L)
print("averages over rows:")
for row in range(L.shape[0]):
print(L[row,:].mean())
print("averages over columns:")
for column in range(L.shape[1]):
print(L[:,column].mean())
# just a test to create, e.g., a 3x5 Matrix:
L = np.linspace((1,11, 7),
(5,15, 11),
9)
print(L)
[[ 1. 11. 7. ]
[ 1.5 11.5 7.5]
[ 2. 12. 8. ]
[ 2.5 12.5 8.5]
[ 3. 13. 9. ]
[ 3.5 13.5 9.5]
[ 4. 14. 10. ]
[ 4.5 14.5 10.5]
[ 5. 15. 11. ]]
# just a test to create a one-line vector:
L = np.linspace(1, 5, 9) # 1=start, 5=stop, 9=number of steps
print(L)
[1. 1.5 2. 2.5 3. 3.5 4. 4.5 5. ]
Exercise 2Permalink
Given the NumPy array A
, that is defined as follows:
A = np.array([np.arange(0,3,1)]*5,dtype='float16')
Write a script that
- prints out its shape.
- prints out all entries of column 1 and 2, separately and at once.
- overwrites the values of column 1 by the sum of the values of columns 2 and 3.
- replaces the entry of row 1, column 3 with its exponentiation with the factor 2 (Hint:
x**2
). - replaces the entry of row 2, column 3 with the square root (
np.sqrt(value)
) of the entry of row 2, column 0 . - prints out all entries with even index values of column 3.
- calculates the average and standard deviation of all entries of column 3.
# Your solution 2.1 here:
A:
[[0. 1. 2.]
[0. 1. 2.]
[0. 1. 2.]
[0. 1. 2.]
[0. 1. 2.]]
A.shape: (5, 3)
Toggle solution
# Solution 2.1:
A = np.array([np.arange(0,3,1)]*5,dtype='float16')
print("A:")
print(A)
print(f"A.shape: {A.shape}")
# Your solution 2.2 here:
[0. 0. 0. 0. 0.]
[1. 1. 1. 1. 1.]
[[0. 1.]
[0. 1.]
[0. 1.]
[0. 1.]
[0. 1.]]
Toggle solution
# Solution 2.2:
print(A[:, 0])
print(A[:, 1])
print(A[:, 0:2])
# Your solution 2.3 here:
[[3. 1. 2.]
[3. 1. 2.]
[3. 1. 2.]
[3. 1. 2.]
[3. 1. 2.]]
Toggle solution
# Solution 2.3:
A[:,0] = A[:,1] + A[:,2]
print(A)
# Your solution 2.4 and 2.5 here:
4.0
1.732
[[3. 1. 4. ]
[3. 1. 1.732]
[3. 1. 2. ]
[3. 1. 2. ]
[3. 1. 2. ]]
Toggle solution
# Solution 2.4 and 2.5:
A[0,2] = A[0,2]**2
A[1,2] = np.sqrt(A[1,0])
print(A[0,2])
print(A[1,2])
print(A)
# Your solution 2.6 here:
[4. 2. 2.]
Toggle solution
# Solution 2.6:
print(A[0:5:2, 2]) # all entries with even index of column 3
# Your solution 2.7 here:
the result: 2.345703125 +/- 0.83349609375
Toggle solution
# Solution 2.7:
print(f"the result: {A[:,2].mean()} +/- {A[:,2].std()}")
# we can also round the numbers (unfortunately, my
# Jupyter Notebook produces some error here; in your
# Spyder Editor it should work correctly)
# e.g. round(2.65789, 2) means: round the 2.65789 to a
# number with 2 floating point numbers (here to: 2.66)
print(f"{round(A[:,2].mean(), 2)} +/- {round(A[:,2].std(),2)}")
2.349609375 +/- 0.830078125
Exercise 3Permalink
- Generate a Numpy array
time
ranging from 0 to 5 in steps of 0.1. - Calculate the exponential values y=etime (Hint:
np.exp(array)
). - Copy your created
y
into another variable called, e.g.,y_noisy
. - Add some noise to
y_noisy
by addingnp.random.randn(len(time))*10
. - Import the Gaussian 1D filter function from the scipy ndimage package:
from scipy.ndimage import gaussian_filter1d
If the
scipy
module is missing, please install it. Please inform yourself about this function on docs.scipy.org ꜛ. - Apply the Gaussian filter to the noisy signal (
gaussian_filter1d(y_noisy, sigma)
)- with
sigma = 3
- with
sigma = 6
- with
(again, save your results into new variables, e.g., y_denoised_3
and y_denoised_6
)
# Your solution here:
Toggle solution
# Solution 3:
import numpy as np
from scipy.ndimage import gaussian_filter1d
# create data arrays:
time = np.arange(0,5, 0.1)
y = np.exp(time)
# add some noise:
y_noisy = y.copy()
y_noisy = y_noisy + np.random.randn(len(time))*10
# apply filters:
y_denoised_3 = gaussian_filter1d(y_noisy, 3)
y_denoised_6 = gaussian_filter1d(y_noisy, 6)
Shallow vs. Deep CopyPermalink
Also for NumPy arrays account the shallow and deep copy rules we have learned before:
# shallow copy only:
array_1 = np.arange(0,3,1) # create the first array_1
array_2 = array_1 # create another array by
# shallow copying array_1
print("array_2 after shallow copying array_1:")
print("array_1:", array_1, "array_2:", array_2)
array_2 after shallow copying array_1:
array_1: [0 1 2] array_2: [0 1 2]
# modify the shallow copy:
array_2[1] = -7
print("array_2 modified after shallow copying array_1:")
print('array_1:', array_1, 'array_2:', array_2)
array_2 modified after shallow copying array_1:
array_1: [ 0 -7 2] array_2: [ 0 -7 2]
Note, that also array_1
has been modified, too!
# deep copy:
array_2 = array_1.copy() # deep copy in NumPy
array_2[1] = 20
print("array_2 modified after deep copying array_1:")
print('array_1:', array_1, 'array_2:', array_2)
array_2 modified after deep copying array_1:
array_1: [ 0 -7 2] array_2: [ 0 20 2]
A deep copy with NumPy can be achieved via the following commands:
array.copy()
np.copy(array)